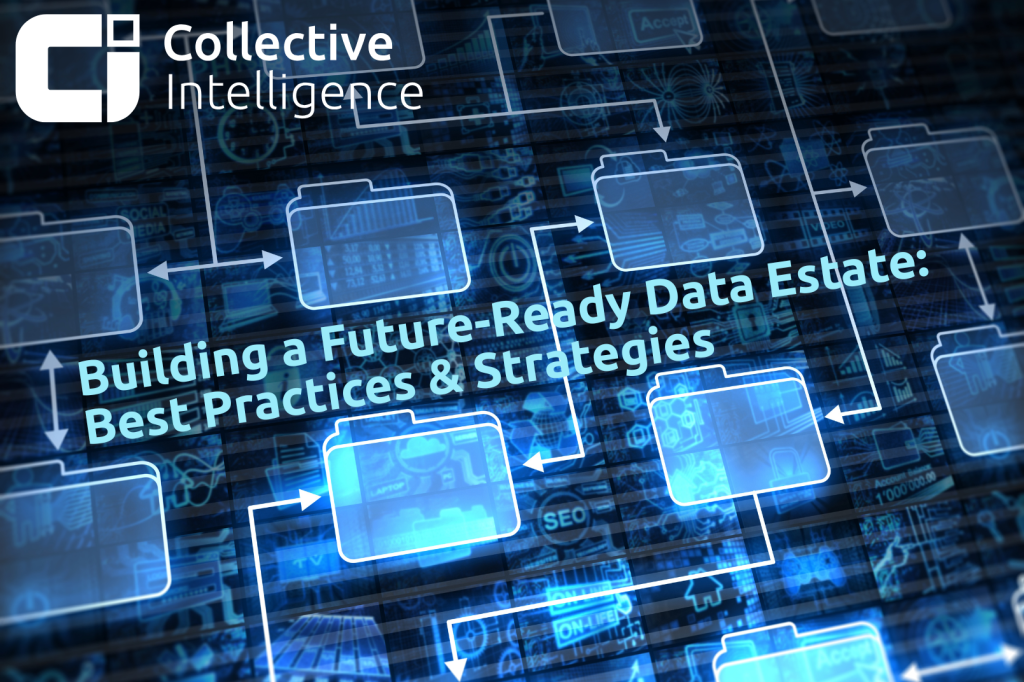
Have you ever wondered how some companies seem to effortlessly manage and leverage vast amounts of data? Meanwhile, others struggle to keep up. In contrast, imagine a bustling city where each piece of data is a building block. The city’s success depends on how well these blocks are organized and utilized. Businesses consider a well-designed future-ready data estate essential for effective modern data management. Below you will discover critical elements and strategies for building a future-ready data estate.
Key Components of a Data Estate
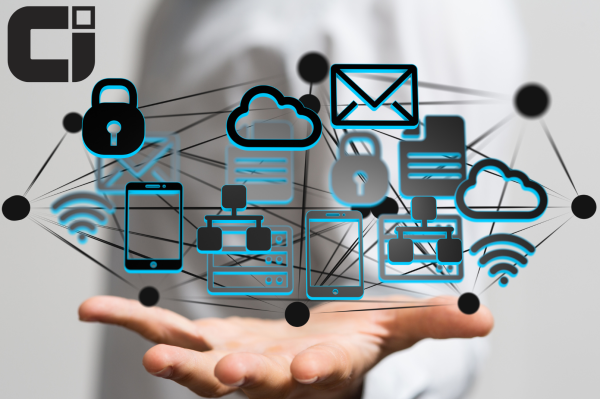
To begin with, several key components are involved in building a robust data estate:
Data Sources
- Business Applications: ERP systems, CRM systems, and other enterprise applications generate valuable data for organizations.
- Legacy Systems: Many organizations still rely on legacy systems for critical business operations. These systems often contain valuable historical data that needs to be integrated into the modern data estate. Integrating legacy systems can be challenging due to outdated technology and data formats, but it is essential for a comprehensive data strategy.
- IoT Devices: Data is collected by Internet of Things (IoT) devices from various sensors and connected equipment. These devices provide real-time insights for businesses.
- External Data Sources: Market data, social media feeds, and public data sets enrich internal data assets.
Storage Solutions
- On-Premises Storage: Traditional data centers and local servers offer direct control over data within physical infrastructure.
- Cloud Storage: Solutions such as Microsoft Azure, AWS, and Google Cloud offer scalability and flexibility without large infrastructure investments.
- Hybrid Storage: On-premises and cloud storage approaches are combined here. This offers the benefits from both storage methodologies.
Data Processing and Analytic Tools
- ETL Tools: Extract, Transform, Load tools integrate data from various sources, transforming raw data into usable formats for analysis.
- Data Warehouses: Central repositories for structured data storage are served here. Data warehouses organize information for efficient querying and reporting.
- Data Lakes: These repositories hold vast amounts of raw data in native format. Data lakes accommodate structured, semi-structured, and unstructured information.
- Analytics Platforms: Comprehensive analysis is enabled by tools like Microsoft Power BI, Tableau, and Databricks. Analytics platforms transform raw data into visual insights. These visualizations simplify complex data, revealing patterns and trends that might be missed in raw formats, leading to a deeper understanding and better use of data. Furthermore, visual insights foster collaboration, enhance retention of information, and can even improve productivity.
Data Quality Management
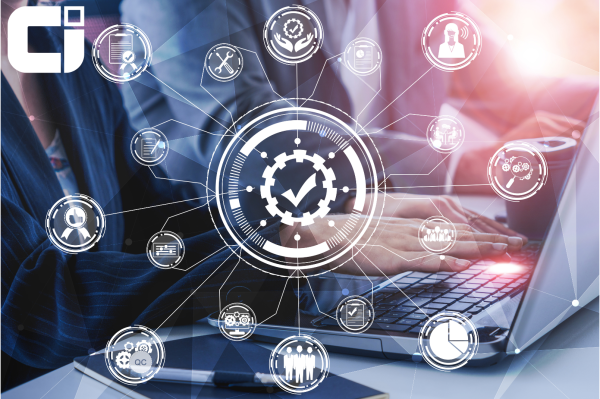
Importantly, maintaining high-quality data is fundamental to deriving value from your data estate. Without proper quality controls, even the most sophisticated analytics tools will produce unreliable results—remember the adage “garbage in, garbage out.” Implementing comprehensive data quality management ensures your organization can trust the insights generated from your data assets.
Quality Assurance Processes
- Data Profiling: Examine data to understand its structure, content, and quality. Use statistical analysis to identify anomalies, outliers, and patterns that may indicate quality issues.
- Standardization: Establish consistent formats for names, addresses, dates, and other common data elements across all systems and sources.
- Deduplication: Identify and eliminate duplicate records that can skew analytics and waste storage resources. Implement matching algorithms to detect similar records that might represent the same entity.
Monitoring and Maintenance
- Quality Metrics: Define key performance indicators (KPIs) for data quality, such as completeness, accuracy, consistency, timeliness, and validity.
- Automated Validation: Implement real-time validation rules to catch errors at the point of data entry or ingestion.
- Data Stewardship: Assign responsibility for ongoing quality monitoring and issue resolution to designated data stewards who understand both the technical and business context of the data.
Remediation Strategies
- Cleansing Workflows: Establish automated processes to correct common errors, standardize formats, and enrich data with missing elements.
- Root Cause Analysis: When quality issues arise, trace them to their source to prevent recurrence rather than simply fixing the symptoms.
- Feedback Loops: Create mechanisms for users to report data quality issues they encounter, closing the loop between data consumers and producers.
Best Practices for Designing a Data Estate
Moving forward, to build a robust future-ready data estate, consider the following best practices:
Scalability
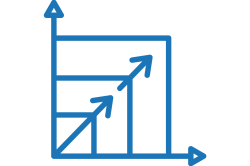
- Design the data estate to handle growing data volumes and increased demand.
- Implement scalable data processing tools for efficient management of large datasets.
- Ensure infrastructure can grow alongside business requirements.
Flexibility
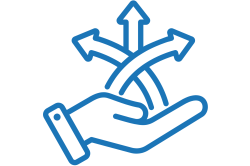
- Choose storage solutions that support various data types and formats, such as structured, semi-structured, and unstructured.
- Use flexible integration tools that connect to multiple sources.
- Ensure systems can adapt to changing business requirements over time.
Cost-Effectiveness
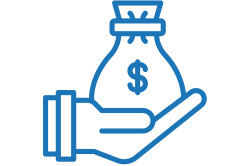
- Optimize storage costs by combining on-premises and cloud solutions.
- Store frequently accessed data in the cloud and less critical data on-premises.
- Implement data archiving and tiering strategies for better cost management. Organizations should allocate resources based on data importance and usage.
Data Governance
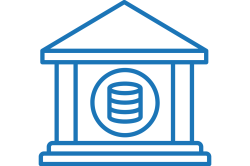
- Establish governance policies to ensure quality, security, and compliance.
- Clearly define data ownership, access controls, and stewardship roles.
- Implement cataloging tools to maintain an inventory of data assets. Teams should track metadata to improve discoverability and understanding across the organization.
Security and Compliance
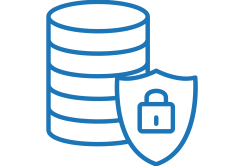
- Protect sensitive information with robust security measures and protocols.
- Utilize encryption, access controls, and regular security audits.
- Ensure compliance with regulations like GDPR, HIPAA, and CCPA. Organizations must implement appropriate data protection practices across all departments.
Partnering with Collective Intelligence
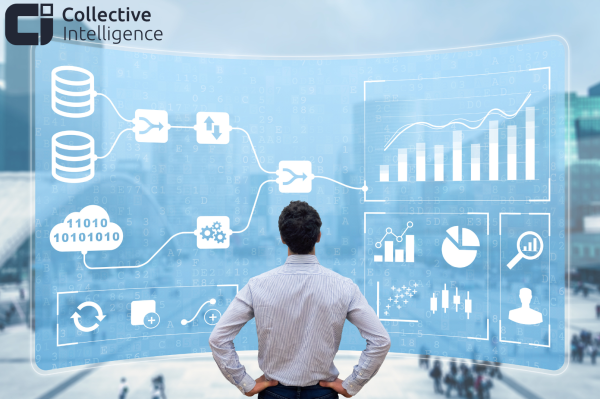
Collective Intelligence is at the forefront of helping companies build future-ready data estates. By leveraging industry-leading tools like Microsoft Fabric and Databricks, we ensure that your data infrastructure is not only scalable but also adaptable to future technological advancements. Our holistic approach includes setting up modern data platforms, integrating data into OneLake, and establishing the Medallion Architecture using Data Warehouses and Lakehouses. This ensures seamless data management and real-time analytics, empowering your business to stay ahead in the competitive landscape.
- Scalability and Adaptability: Build systems that grow with technological advancements and accommodate evolving business needs.
- Modern Data Platforms: Implement innovative platforms for efficient data management and optimized workflows.
- Integration with OneLake: Seamlessly connect data sources to OneLake for unified access across organizations.
- Medallion Architecture: Utilize Data Warehouses and Lakehouses for comprehensive management of both structured and unstructured data.
- Real-Time Analytics: Enable immediate data processing for timely insights and informed decision-making.
Real-World Examples
To illustrate these concepts, let’s examine some real-world examples:
Healthcare: Mayo Clinic
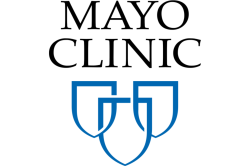
Mayo Clinic implemented a hybrid data estate to manage patient data from various sources, including electronic health records (EHRs), IoT devices, and research databases. This approach enabled them to integrate and analyze data more effectively, leading to improved patient outcomes and operational efficiency.
Finance: Goldman Sachs
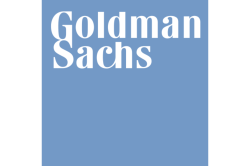
Goldman Sachs built a scalable data estate using cloud storage and advanced analytics tools. This allowed them to process and analyze large volumes of financial data in real-time, enhancing their risk management and trading strategies.
Retail: Walmart
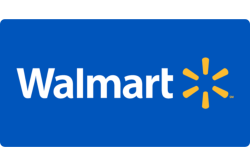
Walmart leveraged a data lake to store and analyze vast amounts of customer data from their online and physical stores. This enabled them to gain insights into customer behavior, optimize inventory management, and personalize marketing campaigns.
Conclusion
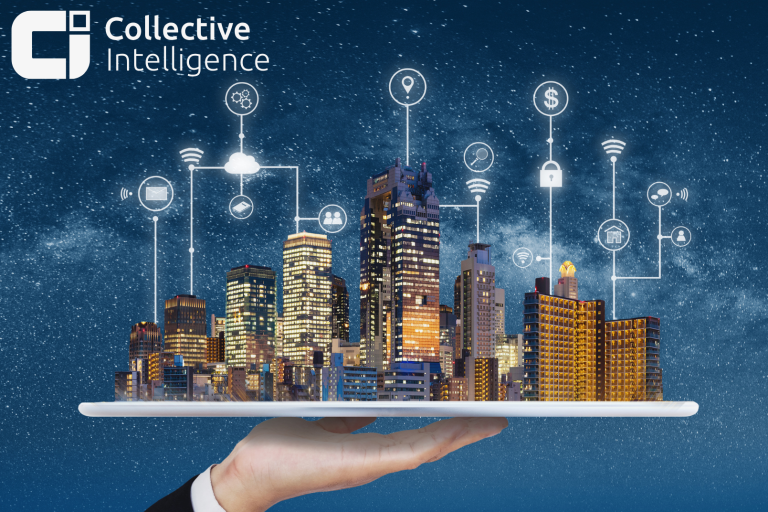
In today’s data-driven business landscape, a robust data estate is no longer optional—it is essential for competitive advantage and innovation. Building such an infrastructure requires careful attention to key components: diverse data sources, flexible storage solutions, and powerful processing tools, all supported by thoughtful governance and security practices.
The organizations that thrive in the digital economy are those that treat their data as a strategic asset, investing in scalable architecture that can evolve alongside changing business needs and technological advancements. As demonstrated by industry leaders like Mayo Clinic, Goldman Sachs, and Walmart, a well-designed data estate creates tangible business value through improved decision-making, operational efficiency, and customer insights.
Whether you are embarking on a comprehensive data transformation or looking to optimize your existing infrastructure, the principles outlined in this guide provide a foundation for creating a future-ready data estate. Remember that building a future-ready data estate is not a one-time project but an ongoing journey of continuous improvement and adaptation.
By embracing best practices in data management today, you position your organization to harness the full potential of your data assets tomorrow, turning information into actionable intelligence that drives business growth and innovation.
To learn more about our services and how we can assist you in building a future-ready data estate, please visit our page or schedule a virtual meeting.